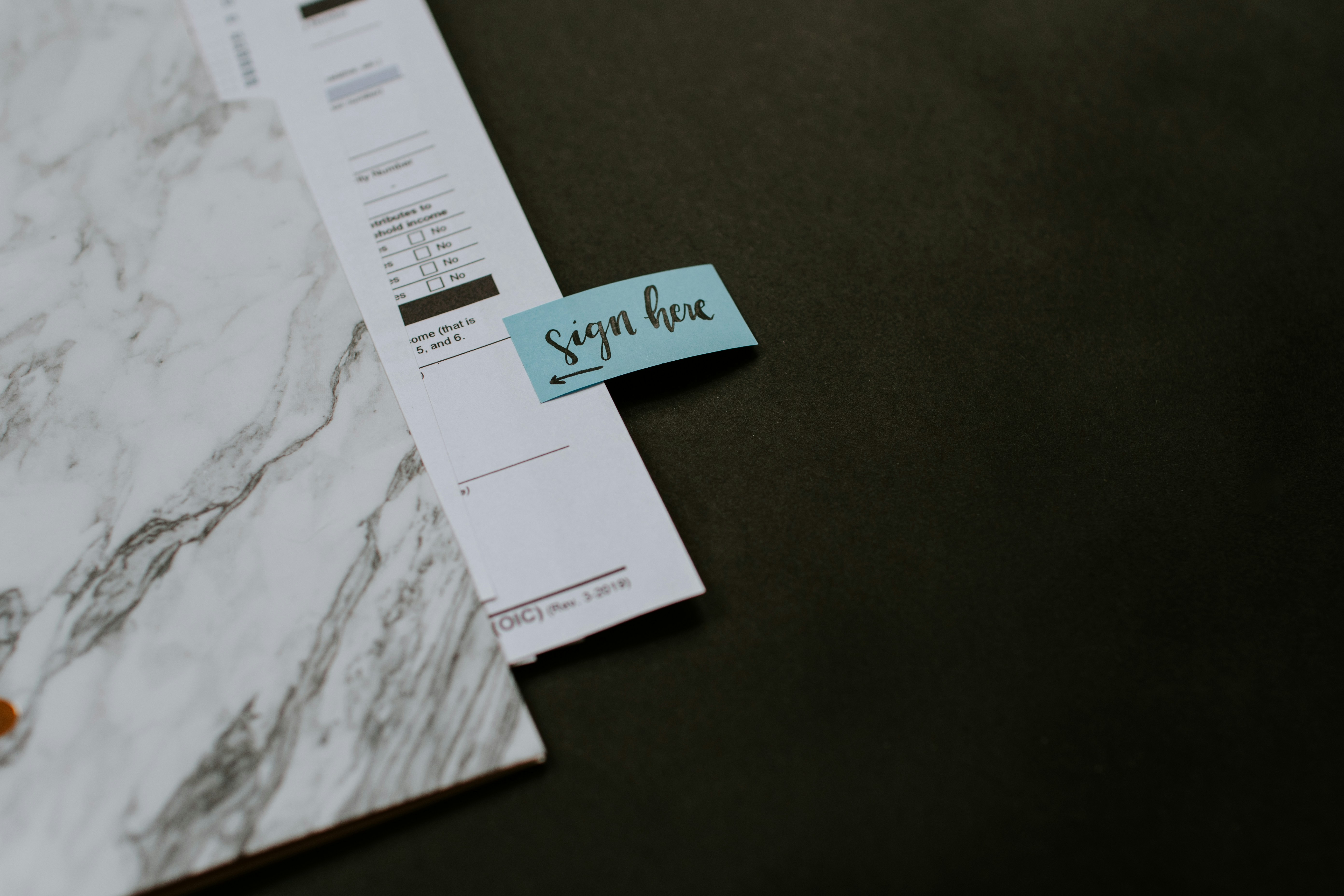
Contract vs Permanent Biotech Jobs: Which Pays Better in 2025?
In the ever-advancing world of life sciences, biotechnology has emerged as one of the fastest-growing and most impactful sectors in the UK. From gene editing and regenerative medicine to synthetic biology and vaccine development, British biotech organisations are at the forefront of scientific innovation. With this growth comes a high demand for skilled professionals—scientists, data analysts, regulatory affairs specialists, bioprocess engineers, and more—who can help bring cutting-edge treatments and products to market. However, as the biotechnology landscape in the UK continues to expand, so too do the ways in which professionals choose to work. Beyond traditional permanent employment, many biotech specialists are exploring the world of contracting, which can take the form of day‑rate consulting or fixed-term contracts (FTC). Each path promises unique advantages and disadvantages, from pay rates and benefits packages to career development and job security. This article offers a comprehensive guide on contract vs. permanent biotech jobs in 2025. We will explore how the UK biotech sector is shaping up, break down the different types of employment arrangements, and present sample take‑home pay scenarios for each. Our goal is to empower you with the knowledge needed to make an informed decision that aligns with both your financial aspirations and long-term career goals within the vibrant and evolving biotech industry.